tensorflow 2.0 深度学习(第二部分 part1)
" class="reference-link">
日萌社
人工智能AI:Keras PyTorch MXNet TensorFlow PaddlePaddle 深度学习实战(不定时更新)
tensorflow 2.0 深度学习(第一部分 part1)
tensorflow 2.0 深度学习(第一部分 part2)
tensorflow 2.0 深度学习(第一部分 part3)
tensorflow 2.0 深度学习(第二部分 part1)
tensorflow 2.0 深度学习(第二部分 part2)
tensorflow 2.0 深度学习(第二部分 part3)
tensorflow 2.0 深度学习 (第三部分 卷积神经网络 part1)
tensorflow 2.0 深度学习 (第三部分 卷积神经网络 part2)
tensorflow 2.0 深度学习(第四部分 循环神经网络)
tensorflow 2.0 深度学习(第五部分 GAN生成神经网络 part1)
tensorflow 2.0 深度学习(第五部分 GAN生成神经网络 part2)
tensorflow 2.0 深度学习(第六部分 强化学习)
神经网络
" class="reference-link">感知机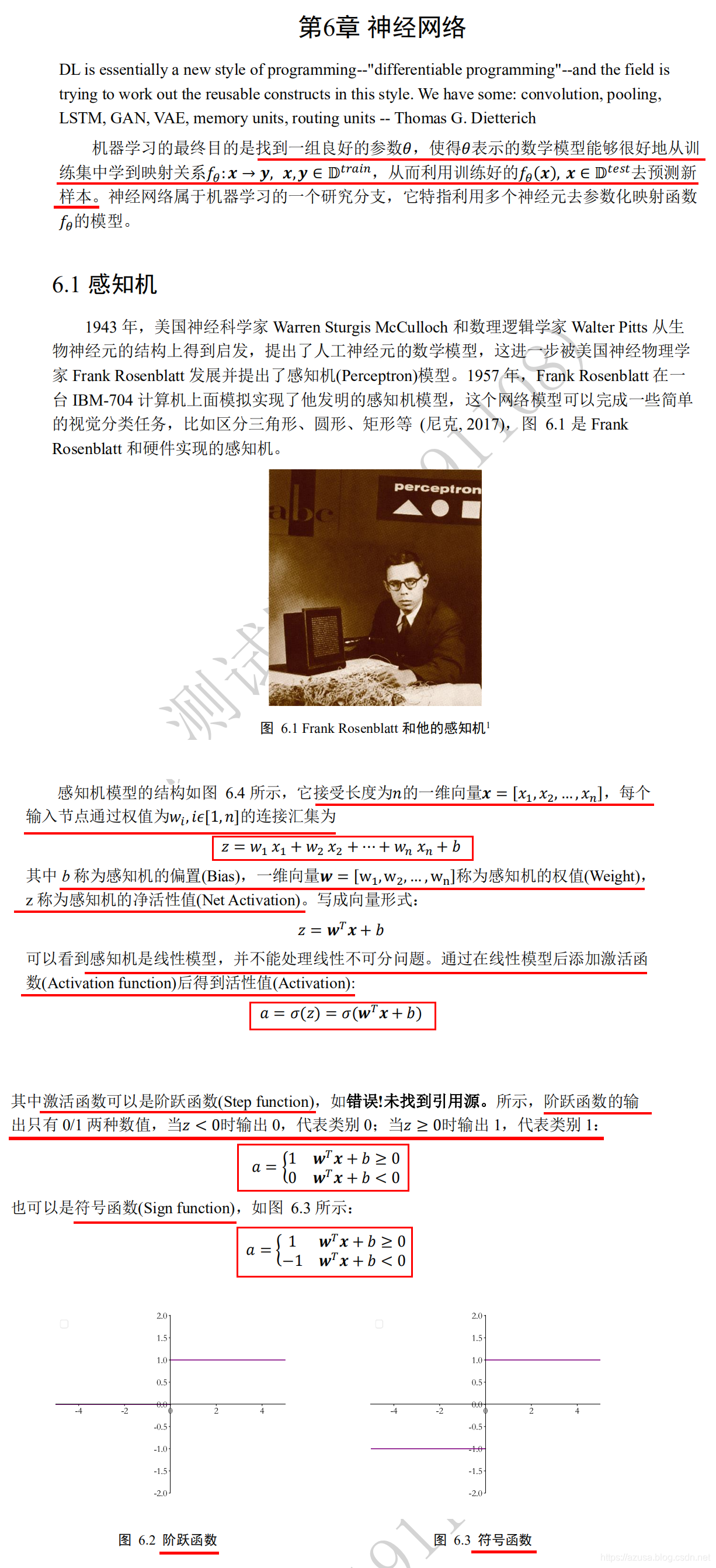
全连接层
import tensorflow as tf
from tensorflow import keras
x = tf.random.normal([2, 3])
model = keras.Sequential([
keras.layers.Dense(2, activation='relu'),
keras.layers.Dense(2, activation='relu'),
keras.layers.Dense(2)
])
#通过 build 函数完成内部张量的创建,其中None为任意的 batch 数量,3为输入特征长度
model.build(input_shape=[None, 3])
model.summary()
for p in model.trainable_variables:
print(p.name, p.shape)
前向传播、反向传播、误差函数
import tensorflow as tf
from tensorflow import keras
from tensorflow.keras import layers
from tensorflow.keras import datasets
import os
x = tf.random.normal([2,28*28]) #[2,784]
w1 = tf.Variable(tf.random.truncated_normal([784, 256], stddev=0.1))
b1 = tf.Variable(tf.zeros([256]))
o1 = tf.matmul(x,w1) + b1 #[2,784]@[784, 256]+[256]
o1
x = tf.random.normal([4,28*28])#[4,784]
fc1 = layers.Dense(256, activation=tf.nn.relu) #[4,784]@[784,256]+[256]= [b, 784] => [b, 256]
fc2 = layers.Dense(128, activation=tf.nn.relu) #[4,256]@[256,128]+[128]= [b, 256] => [b, 128]
fc3 = layers.Dense(64, activation=tf.nn.relu) #[4,128]@[128,64]+[64]= [b, 128] => [b, 64]
fc4 = layers.Dense(10, activation=None) #[4,64]@[64,10]+[10]= [b, 64] => [b, 10]
h1 = fc1(x)
h2 = fc2(h1)
h3 = fc3(h2)
h4 = fc4(h3)
model = layers.Sequential([
layers.Dense(256, activation=tf.nn.relu) ,
layers.Dense(128, activation=tf.nn.relu) ,
layers.Dense(64, activation=tf.nn.relu) ,
layers.Dense(10, activation=None) ,
])
out = model(x)# 256*784+256 + 128*256+128 + 64*128+64 + 10*64+10
os.environ['TF_CPP_MIN_LOG_LEVEL'] = '2'
# x: [60k, 28, 28],
# y: [60k]
(x, y), _ = datasets.mnist.load_data()
# x: [0~255] => [0~1.]
x = tf.convert_to_tensor(x, dtype=tf.float32) / 255. #标准化/归一化
y = tf.convert_to_tensor(y, dtype=tf.int32)
print(x.shape, y.shape, x.dtype, y.dtype)
print(tf.reduce_min(x), tf.reduce_max(x))
print(tf.reduce_min(y), tf.reduce_max(y))
train_db = tf.data.Dataset.from_tensor_slices((x,y)).batch(128) #batch构建批量大小
train_iter = iter(train_db)#iter返回生成器对象
sample = next(train_iter)#next调用生成器返回第一个批量大小的数据
print('batch:', sample[0].shape, sample[1].shape)
# [b, 784] => [b, 256] => [b, 128] => [b, 10]
# [dim_in, dim_out], [dim_out] 第N层权重[输入神经元节点数, 输出神经元节点数]、偏置[输出神经元节点数]
# 隐藏层1张量
w1 = tf.Variable(tf.random.truncated_normal([784, 256], stddev=0.1))
b1 = tf.Variable(tf.zeros([256]))
# 隐藏层2张量
w2 = tf.Variable(tf.random.truncated_normal([256, 128], stddev=0.1))
b2 = tf.Variable(tf.zeros([128]))
# 隐藏层3张量
w3 = tf.Variable(tf.random.truncated_normal([128, 64], stddev=0.1))
b3 = tf.Variable(tf.zeros([64]))
# 输出层张量
w4 = tf.Variable(tf.random.truncated_normal([64, 10], stddev=0.1))
b4 = tf.Variable(tf.zeros([10]))
lr = 1e-3 #学习率
#构建epoch训练次数
for epoch in range(10): # iterate db for 10
#遍历生成器对象,每次获取每个批量大小的数据
for step, (x, y) in enumerate(train_db): # for every batch
# x:[128, 28, 28]
# y: [128]
# [b, 28, 28] => [b, 28*28] 展平为 (批量大小,行*列)
x = tf.reshape(x, [-1, 28*28])
#构建梯度记录环境
with tf.GradientTape() as tape: # tf.Variable
# x: [b, 28*28]
# 隐藏层1前向计算,[b, 28*28] => [b, 256]
h1 = x@w1 + tf.broadcast_to(b1, [x.shape[0], 256])
h1 = tf.nn.relu(h1)
# 隐藏层2前向计算,[b, 256] => [b, 128]
h2 = h1@w2 + b2
h2 = tf.nn.relu(h2)
# 隐藏层3前向计算,[b, 128] => [b, 64]
h3 = h2@w3 + b3
h3 = tf.nn.relu(h3)
# 输出层前向计算,[b, 64] => [b, 10]
h4 = h3@w4 + b4
out = h4
# compute loss
# out: [b, 10]
# y: [b] => [b, 10] 真实标签one-hot化
y_onehot = tf.one_hot(y, depth=10)
# mse = mean(sum(y-out)^2)
# [b, 10] 均方差mse = mean(sum(y-out)^2) 预测值与真实值之差的平方的平均值
loss = tf.square(y_onehot - out)
# mean: scalar
loss = tf.reduce_mean(loss)
#1.求导,tape.gradient(y,[参数θ])求参数θ相对于y的梯度信息
# dy_dw = tape.gradient(y, [w])
#2.通过tape.gradient(loss,[参数θ])函数求得网络参数θ的梯度信息
# grads = tape.gradient(loss, [w1, b1, w2, b2, w3, b3])
# 根据loss对模型所有参数求导 tape.gradient(loss, model.trainable_variables)
# compute gradients
grads = tape.gradient(loss, [w1, b1, w2, b2, w3, b3, w4, b4])
# print(grads)
# w1 = w1 - lr * w1_grad 优化器规则,根据 模型参数θ = θ - lr * grad 更新网络参数
w1.assign_sub(lr * grads[0])
b1.assign_sub(lr * grads[1])
w2.assign_sub(lr * grads[2])
b2.assign_sub(lr * grads[3])
w3.assign_sub(lr * grads[4])
b3.assign_sub(lr * grads[5])
w4.assign_sub(lr * grads[6])
b4.assign_sub(lr * grads[7])
if step % 100 == 0:
print(epoch, step, 'loss:', float(loss))
#
激活函数(非线性函数)
" class="reference-link">输出层设计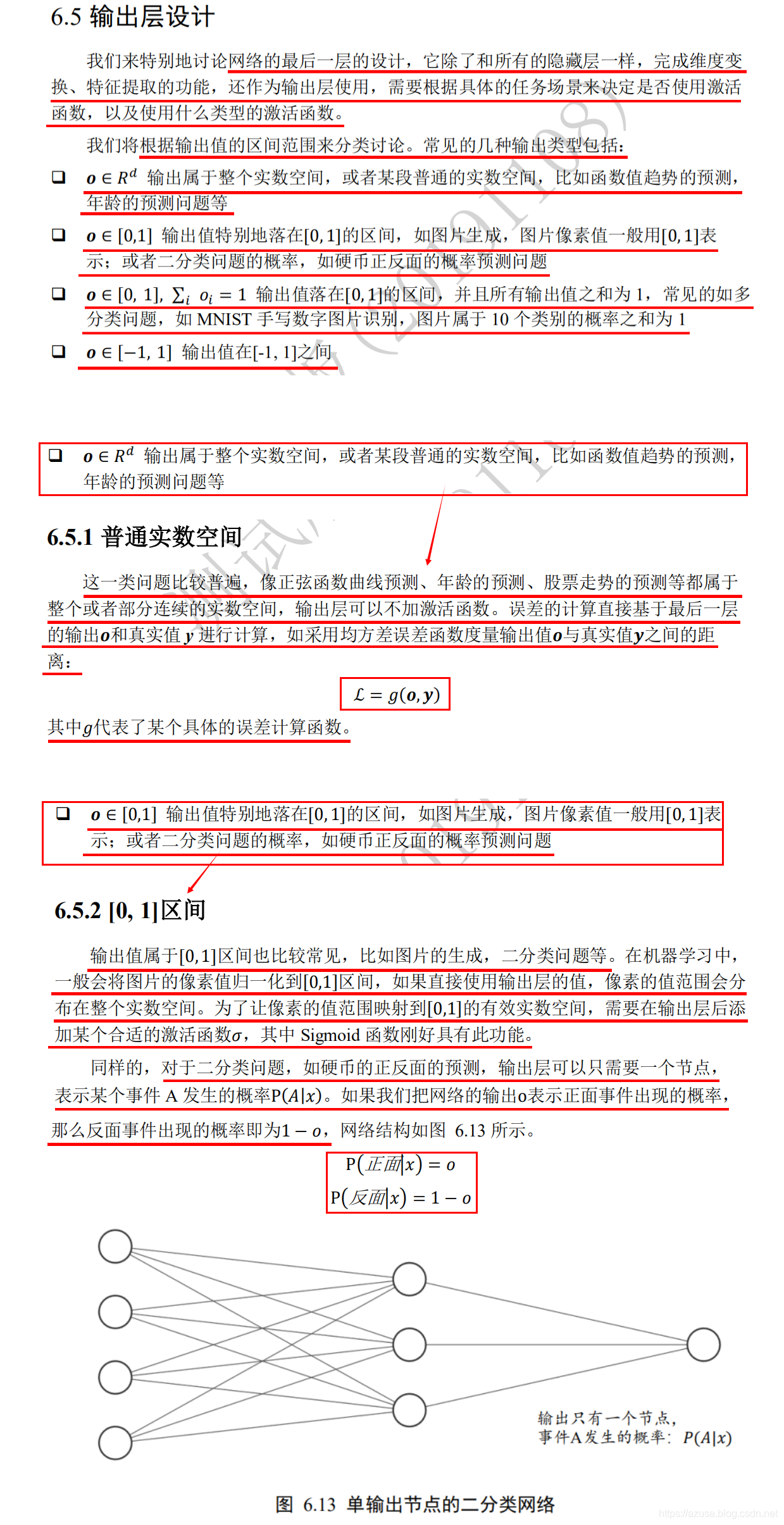
import tensorflow as tf
help(tf.losses.categorical_crossentropy)
查看categorical_crossentropy函数的默认参数列表和使用方法介绍
其中形参默认为from_logits=False,网络预测值y_pred 表示必须为经过了 Softmax函数的输出值。
当 from_logits 设置为 True 时,网络预测值y_pred 表示必须为还没经过 Softmax 函数的变量 z。
from_logits=True 标志位将softmax激活函数实现在损失函数中,便不需要手动添加softmax损失函数,提升数值计算稳定性。
Help on function categorical_crossentropy in module tensorflow.python.keras.losses:
categorical_crossentropy(y_true, y_pred, from_logits=False, label_smoothing=0)
Computes the categorical crossentropy loss.
Args:
y_true: tensor of true targets.
y_pred: tensor of predicted targets.
from_logits: Whether `y_pred` is expected to be a logits tensor. By default,
we assume that `y_pred` encodes a probability distribution.
" class="reference-link">loss误差函数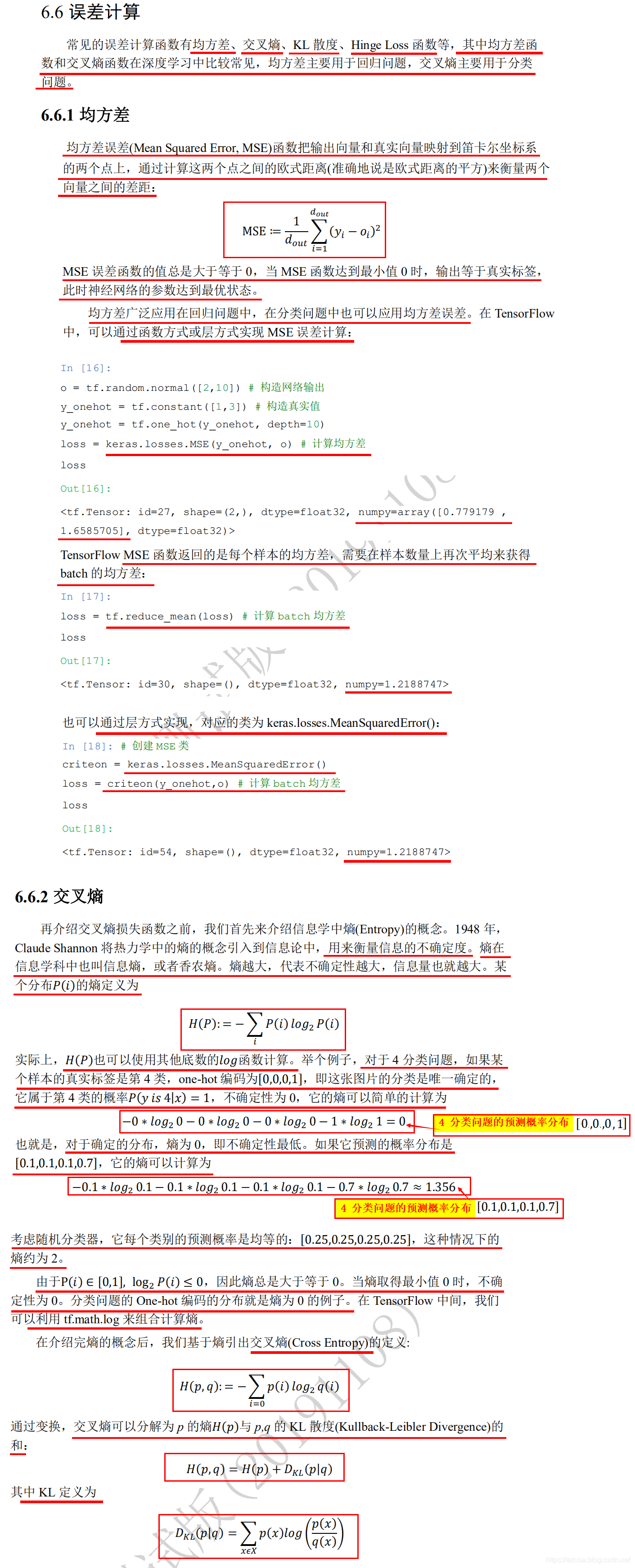
import tensorflow as tf
x=tf.random.normal([1,3])
w=tf.ones([3,2])
b=tf.ones([2])
y = tf.constant([0, 1])
with tf.GradientTape() as tape:
tape.watch([w, b])
logits = tf.sigmoid(x@w+b) #非线性激活函数sigmoid(线性函数x@w+b)
loss = tf.reduce_mean(tf.losses.MSE(y, logits)) #MSE均方差损失函数(y真实值-预测值)
#通过loss对模型参数θ的梯度
grads = tape.gradient(loss, [w, b])
print('w grad:', grads[0])
print('b grad:', grads[1])
import tensorflow as tf
tf.random.set_seed(4323) #随机种子
x=tf.random.normal([1,3])
w=tf.random.normal([3,2])
b=tf.random.normal([2])
y = tf.constant([0, 1])
with tf.GradientTape() as tape:
tape.watch([w, b])
logits = (x@w+b) #线性函数x@w+b
#from_logits默认为from_logits=False,网络预测值y_pred 表示必须为经过了 Softmax函数的输出值。
#当 from_logits 设置为 True 时,网络预测值y_pred 表示必须为还没经过 Softmax 函数的变量z。
loss = tf.reduce_mean(tf.losses.categorical_crossentropy(y, logits, from_logits=True))
#通过loss对模型参数θ的梯度
grads = tape.gradient(loss, [w, b])
print('w grad:', grads[0])
print('b grad:', grads[1])
import tensorflow as tf
from tensorflow import keras
from tensorflow.keras import datasets, layers
import os
a = tf.random.normal([4,35,8]) # 模拟成绩册A
b = tf.random.normal([6,35,8]) # 模拟成绩册B
tf.concat([a,b],axis=0) # 合并成绩册 TensorShape([10, 35, 8])
x = tf.random.normal([2,784])
w1 = tf.Variable(tf.random.truncated_normal([784, 256], stddev=0.1))
b1 = tf.Variable(tf.zeros([256]))
o1 = tf.matmul(x,w1) + b1 #[2,784]@[784, 256]+[256]=[2, 256]
o1 = tf.nn.relu(o1)
o1 #TensorShape([2, 256])
x = tf.random.normal([4,28*28])
# 创建全连接层,指定输出节点数和激活函数
fc = layers.Dense(512, activation=tf.nn.relu)
h1 = fc(x) # 通过fc类完成一次全连接层的计算
vars(fc)
x = tf.random.normal([4,4])
# 创建全连接层,指定输出节点数和激活函数
fc = layers.Dense(3, activation=tf.nn.relu)
h1 = fc(x) # 通过fc类完成一次全连接层的计算
fc.non_trainable_variables
embedding = layers.Embedding(10000, 100) #词汇表单词数量为10000,每个单词的维度为100
x = tf.ones([25000,80])
embedding(x)
z = tf.random.normal([2,10]) # 构造输出层的输出
y_onehot = tf.constant([1,3]) # 构造真实值
y_onehot = tf.one_hot(y_onehot, depth=10) # 真实值one-hot编码 TensorShape([2, 10])
# from_logits=True表示 输出层未使用Softmax函数,故from_logits设置为True
loss = keras.losses.categorical_crossentropy(y_onehot,z,from_logits=True)
loss = tf.reduce_mean(loss) # 计算平均交叉熵损失
loss
# from_logits=True表示 输出层未使用Softmax函数,故from_logits设置为True
criteon = keras.losses.CategoricalCrossentropy(from_logits=True) # 计算平均交叉熵损失
loss = criteon(y_onehot,z) # 计算损失
loss
import tensorflow as tf
y = tf.constant([1, 2, 3, 0, 2])
y = tf.one_hot(y, depth=4) #TensorShape([5, 4])
y = tf.cast(y, dtype=tf.float32)
out = tf.random.normal([5, 4])
# mse = mean(sum(y-out)^2) 均方差mse = mean(sum(y-out)^2) 预测值与真实值之差的平方的平均值
loss1 = tf.reduce_mean(tf.square(y-out))
#tf.norm(a)默认为执行L2范数tf.norm(a. ord=2),等同于tf.sqrt(tf.reduce_sum(tf.square(a)))
loss2 = tf.square(tf.norm(y-out)) / (5*4)
# MSE 函数返回的是每个样本的均方差,需要在样本数量上再次平均来获得 batch 的均方差
# loss = keras.losses.MSE(y_onehot, o) # 计算均方差
# loss = tf.reduce_mean(loss) # 计算 batch 均方差
loss3 = tf.reduce_mean(tf.losses.MSE(y, out))
print(loss1) #tf.Tensor(0.96629584, shape=(), dtype=float32)
print(loss2) #tf.Tensor(0.9662959, shape=(), dtype=float32)
print(loss3) #tf.Tensor(0.96629584, shape=(), dtype=float32)
import tensorflow as tf
help(tf.losses.categorical_crossentropy)
查看categorical_crossentropy函数的默认参数列表和使用方法介绍
其中形参默认为from_logits=False,网络预测值y_pred 表示必须为经过了 Softmax函数的输出值。
当 from_logits 设置为 True 时,网络预测值y_pred 表示必须为还没经过 Softmax 函数的变量 z。
from_logits=True 标志位将softmax激活函数实现在损失函数中,便不需要手动添加softmax损失函数,提升数值计算稳定性。
Help on function categorical_crossentropy in module tensorflow.python.keras.losses:
categorical_crossentropy(y_true, y_pred, from_logits=False, label_smoothing=0)
Computes the categorical crossentropy loss.
Args:
y_true: tensor of true targets.
y_pred: tensor of predicted targets.
from_logits: Whether `y_pred` is expected to be a logits tensor. By default,
we assume that `y_pred` encodes a probability distribution.
神经网络类型
油耗预测实战
#统计数据
sns.pairplot(train_dataset[["Cylinders", "Displacement", "Weight", "MPG"]], diag_kind="kde")
#查看训练集的输入X的统计数据
train_stats = train_dataset.describe()
# MPG Cylinders Displacement Horsepower ... Model Year USA Europe Japan
#count 314.000000 314.000000 314.000000 314.000000 ... 314.000000 314.000000 314.000000 314.000000
#mean 23.310510 5.477707 195.318471 104.869427 ... 75.898089 0.624204 0.178344 0.197452
#std 7.728652 1.699788 104.331589 38.096214 ... 3.675642 0.485101 0.383413 0.398712
#min 10.000000 3.000000 68.000000 46.000000 ... 70.000000 0.000000 0.000000 0.000000
#25% 17.000000 4.000000 105.500000 76.250000 ... 73.000000 0.000000 0.000000 0.000000
#50% 22.000000 4.000000 151.000000 94.500000 ... 76.000000 1.000000 0.000000 0.000000
#75% 28.950000 8.000000 265.750000 128.000000 ... 79.000000 1.000000 0.000000 0.000000
#max 46.600000 8.000000 455.000000 225.000000 ... 82.000000 1.000000 1.000000 1.000000
from __future__ import absolute_import, division, print_function, unicode_literals
import pathlib
import os
import matplotlib.pyplot as plt
import pandas as pd
import seaborn as sns
import tensorflow as tf
from tensorflow import keras
from tensorflow.keras import layers, losses
print(tf.__version__) #2.0.0
os.environ['TF_CPP_MIN_LOG_LEVEL'] = '2'
# 在线下载汽车效能数据集
dataset_path = keras.utils.get_file("auto-mpg.data", "http://archive.ics.uci.edu/ml/machine-learning-databases/auto-mpg/auto-mpg.data")
# 效能(公里数每加仑),气缸数,排量,马力,重量
# 加速度,型号年份,产地
column_names = ['MPG','Cylinders','Displacement','Horsepower','Weight',
'Acceleration', 'Model Year', 'Origin']
raw_dataset = pd.read_csv(dataset_path, names=column_names,
na_values = "?", comment='\t',
sep=" ", skipinitialspace=True)
# MPG Cylinders Displacement Horsepower Weight Acceleration Model Year Origin
#0 18.0 8 307.0 130.0 3504.0 12.0 70 1
#1 15.0 8 350.0 165.0 3693.0 11.5 70 1
#2 18.0 8 318.0 150.0 3436.0 11.0 70 1
#3 16.0 8 304.0 150.0 3433.0 12.0 70 1
#4 17.0 8 302.0 140.0 3449.0 10.5 70 1
dataset = raw_dataset.copy()
# 查看部分数据
dataset.tail()
dataset.head()
dataset
# 统计空白数据,并清除
dataset.isna().sum()
dataset = dataset.dropna()
dataset.isna().sum()
dataset #[392 rows x 8 columns]
# 处理类别型数据,其中origin列代表了类别1,2,3,分布代表产地:美国、欧洲、日本
# 其弹出这一列
origin = dataset.pop('Origin')
# 根据origin列来写入新列
dataset['USA'] = (origin == 1)*1.0
dataset['Europe'] = (origin == 2)*1.0
dataset['Japan'] = (origin == 3)*1.0
dataset.tail()
# MPG Cylinders Displacement Horsepower Weight Acceleration Model Year USA Europe Japan
#0 18.0 8 307.0 130.0 3504.0 12.0 70 1.0 0.0 0.0
#1 15.0 8 350.0 165.0 3693.0 11.5 70 1.0 0.0 0.0
#2 18.0 8 318.0 150.0 3436.0 11.0 70 1.0 0.0 0.0
#3 16.0 8 304.0 150.0 3433.0 12.0 70 1.0 0.0 0.0
#4 17.0 8 302.0 140.0 3449.0 10.5 70 1.0 0.0 0.0
# 切分为训练集和测试集
train_dataset = dataset.sample(frac=0.8,random_state=0) #[314 rows x 10 columns]
test_dataset = dataset.drop(train_dataset.index) #[78 rows x 10 columns]
#统计数据
sns.pairplot(train_dataset[["Cylinders", "Displacement", "Weight", "MPG"]], diag_kind="kde")
#查看训练集的输入X的统计数据
train_stats = train_dataset.describe()
# MPG Cylinders Displacement Horsepower ... Model Year USA Europe Japan
#count 314.000000 314.000000 314.000000 314.000000 ... 314.000000 314.000000 314.000000 314.000000
#mean 23.310510 5.477707 195.318471 104.869427 ... 75.898089 0.624204 0.178344 0.197452
#std 7.728652 1.699788 104.331589 38.096214 ... 3.675642 0.485101 0.383413 0.398712
#min 10.000000 3.000000 68.000000 46.000000 ... 70.000000 0.000000 0.000000 0.000000
#25% 17.000000 4.000000 105.500000 76.250000 ... 73.000000 0.000000 0.000000 0.000000
#50% 22.000000 4.000000 151.000000 94.500000 ... 76.000000 1.000000 0.000000 0.000000
#75% 28.950000 8.000000 265.750000 128.000000 ... 79.000000 1.000000 0.000000 0.000000
#max 46.600000 8.000000 455.000000 225.000000 ... 82.000000 1.000000 1.000000 1.000000
train_stats.pop("MPG")
#train_stats['列值字段名']:该方式为通过使用['列值字段名']访问的是列值,不能直接使用['行值字段名']访问行值。
#如要使用行值字段名访问行值的话,必须先transpose()反转张量的行和列,那么本来的行值字段名和行值都变成了列值字段名和列值,
#本来的列值字段名和列值都变成了行值字段名和行值,那么现在便可以使用['原来的行值字段名']访问原来的行值。
#(8, 9) 表示8行统计指标值,9列特征值 => (9, 8) 表示9行特征值,8列统计指标值
train_stats = train_stats.transpose()
train_stats #train_stats['列值字段名']:可以使用train_stats['count'],不可以使用train_stats['Cylinders']
# count mean std min 25% 50% 75% max
#Cylinders 314.0 5.477707 1.699788 3.0 4.00 4.0 8.00 8.0
#Displacement 314.0 195.318471 104.331589 68.0 105.50 151.0 265.75 455.0
#Horsepower 314.0 104.869427 38.096214 46.0 76.25 94.5 128.00 225.0
#Weight 314.0 2990.251592 843.898596 1649.0 2256.50 2822.5 3608.00 5140.0
#Acceleration 314.0 15.559236 2.789230 8.0 13.80 15.5 17.20 24.8
#Model Year 314.0 75.898089 3.675642 70.0 73.00 76.0 79.00 82.0
#USA 314.0 0.624204 0.485101 0.0 0.00 1.0 1.00 1.0
#Europe 314.0 0.178344 0.383413 0.0 0.00 0.0 0.00 1.0
#Japan 314.0 0.197452 0.398712 0.0 0.00 0.0 0.00 1.0
# 移动MPG油耗效能这一列为真实标签Y
train_labels = train_dataset.pop('MPG') #(314,)
test_labels = test_dataset.pop('MPG') #(78,)
# 标准化数据
def norm(x):
return (x - train_stats['mean']) / train_stats['std']
#>>> train_stats['mean'].shape
#(9,)
#>>> train_stats['mean']
#Cylinders 5.477707
#Displacement 195.318471
#Horsepower 104.869427
#Weight 2990.251592
#Acceleration 15.559236
#Model Year 75.898089
#USA 0.624204
#Europe 0.178344
#Japan 0.197452
#Name: mean, dtype: float64
#>>> train_stats['std'].shape
#(9,)
#>>> train_stats['std']
#Cylinders 1.699788
#Displacement 104.331589
#Horsepower 38.096214
#Weight 843.898596
#Acceleration 2.789230
#Model Year 3.675642
#USA 0.485101
#Europe 0.383413
#Japan 0.398712
#Name: std, dtype: float64
normed_train_data = norm(train_dataset)
normed_test_data = norm(test_dataset)
print(normed_train_data.shape,train_labels.shape) #(314, 9) (314,)
print(normed_test_data.shape, test_labels.shape) #(78, 9) (78,)
class Network(keras.Model):
# 回归网络
def __init__(self):
super(Network, self).__init__()
# 创建3个全连接层
self.fc1 = layers.Dense(64, activation='relu')
self.fc2 = layers.Dense(64, activation='relu')
self.fc3 = layers.Dense(1)
def call(self, inputs, training=None, mask=None):
# 依次通过3个全连接层
x = self.fc1(inputs)
x = self.fc2(x)
x = self.fc3(x)
return x
model = Network()
model.build(input_shape=(None, 9))
model.summary()
optimizer = tf.keras.optimizers.RMSprop(0.001)
train_db = tf.data.Dataset.from_tensor_slices((normed_train_data.values, train_labels.values))
train_db = train_db.shuffle(100).batch(32)
# 未训练时测试
# example_batch = normed_train_data[:10]
# example_result = model.predict(example_batch)
# example_result
train_mae_losses = [] #MAE
test_mae_losses = []
#构建epoch训练次数
for epoch in range(200):
#遍历生成器对象,每次获取每个批量大小的数据
for step, (x,y) in enumerate(train_db):
#构建梯度记录环境
with tf.GradientTape() as tape:
out = model(x)
loss = tf.reduce_mean(losses.MSE(y, out)) #MSE
mae_loss = tf.reduce_mean(losses.MAE(y, out)) #MAE
if step % 10 == 0:
print(epoch, step, float(loss))
#1.求导,tape.gradient(y,[参数θ])求参数θ相对于y的梯度信息
# dy_dw = tape.gradient(y, [w])
#2.通过tape.gradient(loss,[参数θ])函数求得网络参数θ的梯度信息
# grads = tape.gradient(loss, [w1, b1, w2, b2, w3, b3])
# 根据loss对模型所有参数求导 tape.gradient(loss, model.trainable_variables)
grads = tape.gradient(loss, model.trainable_variables)
#优化器规则,根据 模型参数θ = θ - lr * grad 更新网络参数
optimizer.apply_gradients(zip(grads, model.trainable_variables))
train_mae_losses.append(float(mae_loss))
out = model(tf.constant(normed_test_data.values))
test_mae_losses.append(tf.reduce_mean(losses.MAE(test_labels, out)))
plt.figure()
plt.xlabel('Epoch')
plt.ylabel('MAE')
plt.plot(train_mae_losses, label='Train')
plt.plot(test_mae_losses, label='Test')
plt.legend()
# plt.ylim([0,10])
plt.legend()
plt.savefig('auto.svg')
plt.show()
还没有评论,来说两句吧...